In the second of our global equity sector hub discussions, Josep Bori, technology sector hub lead, explains where the team is finding its strongest investment convictions in artificial intelligence and beyond.
Read this article to understand:
- Where company spend is in the artificial intelligence (AI) lifecycle
- The importance of the broader background to understand the bulk of tech and IT spend
- Our approach to investing through the current turbulence
The public release of OpenAI’s ChatGPT in late 2022 started a consumer craze, with over 100 million active users by January 2023. Since then, equity investing seems to have been all about generative AI, from the so-called “Magnificent Seven” to DeepSeek. Given all the hype and recent market upheaval, it’s important to take a step back and look more broadly at where the big IT money is being spent, today and for the long term.
As Josep Bori, technology sector hub lead analyst at Aviva Investors, explains in this article, our tech sector hub experts have broken down the AI lifecycle to identify the key phases where spending is concentrated. They have also found investment ideas across a wider set of trends, from continued cloud adoption to analytics and big data.
And through their regular meetings with our other research sector hubs, and the consumer hub in particular, they have analysed the firms beginning to use AI in business, as well as broader trends like the shift in advertising spend towards social media.
But the tech sector also faces risks and challenges. It is important to invest carefully through the turbulence. It is also worth keeping an eye on the emerging technologies that could suddenly take off if a breakthrough were achieved.
The AI lifecycle: follow the money
Understanding how the training and inference of AI models work can uncover three areas where spending happens in the supply chain, which informs where the investment opportunities may be.
First, the pre-training phase exposes the model to a massive amount of data and teaches it basic skills. That creates a foundational model, like ChatGPT. Typically, those models are built by hyper-scalers like AWS or Alphabet. The more data fed in, and the more chips used to process them, the bigger and more effective the model is, which has implications on hyper-scalers’ investments in chips and data centres (see Figure 1).
Figure 1: AI pre-training
Dataset
Preprocessing
Pre-training
General knowledge
Lots of data
Heavy compute
Months to train
Very expensive
Foundational model
Source: Aviva Investors, March 2025.
The second phase requiring investment is post-training. This consists in feeding a foundational model a smaller set of additional data on a specific area and adding human feedback to improve answers. This produces a “specialist” version that delivers better results in a narrow field. For instance, a model can be trained as a chatbot in customer support (see Figure 2).
Figure 2: AI post-training
Foundational model
Post-training
Domain specific data
Human reinforcement learning
Lighter compute
Quicker/cheaper
Use case/domain specific model
Source: Aviva Investors, March 2025.
Thanks to the knowledge shared by our consumer sector hub, we have insight into consumer companies’ challenges and plans to adopt AI. That also allows us to assess the firms that stand to benefit the most when the spending starts shifting to enterprise deployment.
SaaS providers can leverage best practice and offer pre-trained models
Large organisations with IT resources and sensitive datasets, like pharma companies, might licence a model and train it with their own data on a private cloud. Others might use a software-as-a-service (SaaS) solution adapted to their specific needs. As some functionalities are standard across an industry, SaaS providers can leverage best practice and offer pre-trained models. Companies from Salesforce to SAP, Palantir and Microsoft are exploring these “agentic” platforms.
The third investment phase is improving the model’s answers once in use, which is known as reasoning or test-time compute (see Figure 3). AI developers have found that asking models to finetune their answers through multiple iterations led to better responses. As a result, many have automated this process over the last 12 to 18 months. This achieves better results but requires more computing power to remain fast enough to be useful. When a client is on the line, they cannot be asked to wait several minutes for the model to figure out the best answer.
Figure 3: AI test-time compute (inference)
Question
Trained model
Reasoning a.k.a. test-time compute
Faster/low latency
Increasingly compute heavy
Less expensive
Tree of answers under consideration
Answering
Source: Aviva Investors, March 2025.
AI technology has reached its current stage by evolving over the last five to ten years, with different levels of investment intensity (see Figure 4). Before the launch of ChatGPT, most spending was focused on building foundational models, led by Alphabet and start-ups like DeepMind, Hugging Face, OpenAI, Stability AI and Cohere.1
We believe we are now entering a commercial expansion phase
As ChatGPT made generative AI mainstream, a commercial exploration phase started, with most cloud hyper-scalers and a new wave of AI start-ups, such as Anthropic, Mistral and Moonshot AI, developing large-language models for specific use cases or industries, and traditional enterprises running pilots and trials.2
We believe we are now entering a commercial expansion phase, in which enterprises go beyond those pilots and start investing in further business automation using AI.3
Figure 4: Global enterprise IT spending by customer category, 2015-2028E ($ millions)
Source: Aviva Investors, Gartner, Bloomberg. Data as of March 17, 2025.
Again, some companies might want to adopt this using their own hardware, while others might hire capacity from a cloud provider. That means the adoption of AI will drive a lot of IT spending, both in traditional, owned servers and systems and, increasingly, in cloud services.
AI adoption therefore creates a wide array of investment opportunities, from semiconductors and the tools to build them to SaaS companies, and IT services firms like Accenture, Tata, Infosys, and Capgemini because organisations will need help to implement AI.
Looking at the bigger tech picture
In fact, many themes are continuing to play out beyond AI. And to mitigate the risk of the AI story not playing out, we want to diversify into areas where it is not the primary driver.
Figure 5 shows the key topics we watch within the hub. A few big themes like artificial intelligence, cloud computing, semiconductor technology are driving a lot of our investment ideas. And what we do is try to understand how companies are positioned on these.
Figure 5: The tech sector hub thematic view
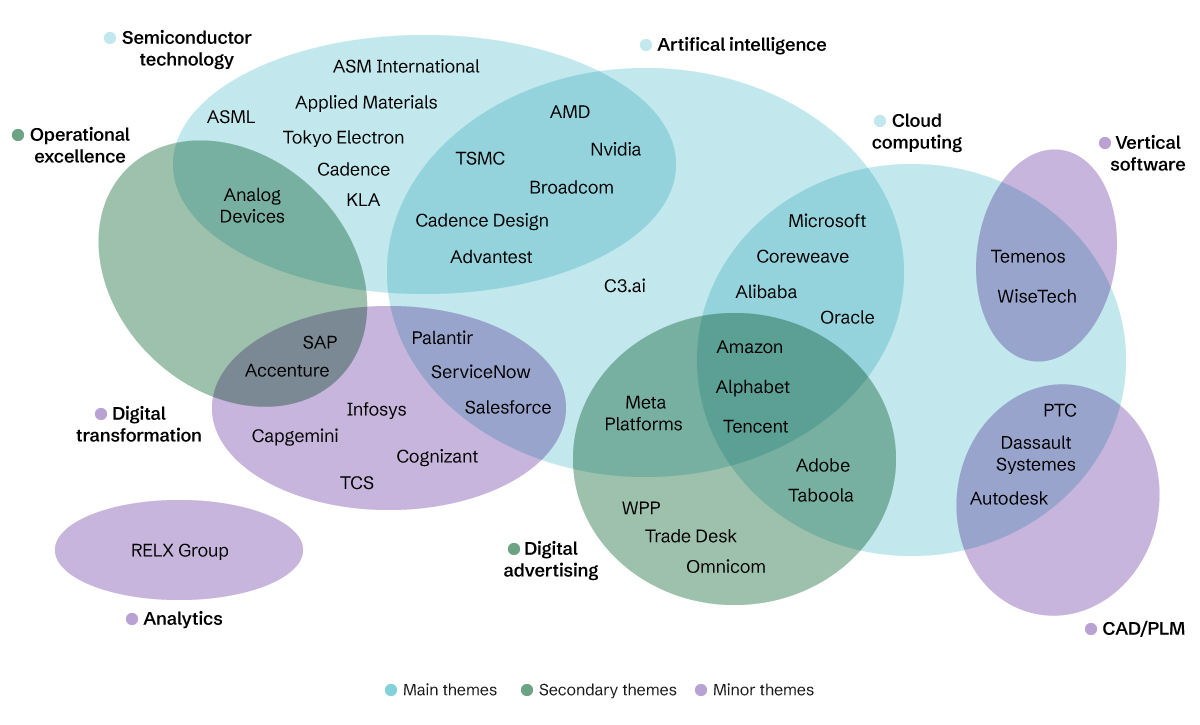
Source: Aviva Investors, March 2025.
For instance, even though Meta also bets very hard on AI, its main theme is that it is a beneficiary of the transition towards digital advertising. It is a large player in a sector that is going through a structural shift from money being spent on TV, magazines and street signs to digital spending.
We hear about consumer goods companies rebalancing their budgets towards social media influencers and platforms
Thanks to our discussions with the consumer sector hub, in addition to the interesting trend towards retail media, we hear about consumer goods companies rebalancing their budgets towards social media influencers and platforms like Instagram or TikTok.4,5
Cloud computing adoption and the digital transformation are other long-running themes that continue to drive tech spending, with some overlap into AI. And we see potential in more niche areas like big-data analytics, virtual and augmented reality, and computer-aided design (CAD) and product lifecycle management (PLM).
Challenges ahead
Of course, there are always risks, and the current climate is particularly turbulent.
US government policies, from tariffs to geopolitics, are generating uncertainty around supply chain security, meaning some spending will move to different places, and some may be cut back (see House view: 2025 Outlook).6
The hyper-scalers’ budget size and long-term investment horizons insulate them somewhat from this environment, but widespread corporate adoption of AI could be much more affected. If a firm’s core business risks being affected by tariffs or geopolitical developments, it may hold back on the rollout of such projects.
Policy might settle at some point, but the constant changes of direction of the past few months make it challenging to find a balance between defensive and growth positions, and to get clarity on potential beneficiaries.
AI adoption also presents risks from a technology standpoint, as it remains to be proven. The foundational models are impressive, but if companies roll out a model to improve their processes, it needs to work well and avoid the extra cost of constant fixes.
Some business processes are more AI-ready than others. For instance, call centres are already set up to minimise cost, by having junior agents answer the bulk of calls and handing over the most complex cases to senior agents. Even without perfect technology, it’s easy to imagine a transition to AI answering most calls and handing complex cases to a human. In contrast, firms are unlikely to take chances with their financial reports or in the medical space.
Depending on how the technology evolves, the risk is that it doesn't work well enough and most companies decide not to invest. The next couple of years will be crucial to see whether these solutions start to deliver. We will carefully invest in related stocks, ready to detect any sign that AI isn’t – or is – working.
Investing through turbulence7
Despite the uncertainty, hyper-scalers’ plans and the broader picture mean we have strong conviction in names like NVIDIA, TSMC and Broadcom.8
When the market is temporarily in risk-off mode, these stocks will fall
We are fully aware that, when the market is temporarily in risk-off mode, these stocks will fall. But we see that short-term volatility as an opportunity to add exposure, because we think these are top-quality companies and very well positioned to benefit from AI adoption in the long run. Of course, as discussed, the risk is that AI adoption gets derailed after a few years of trying, and we remain watchful.
Another core holding for us is Meta, which we like for two reasons. One is because it adds some diversification as the main theme underpinning it is digital advertising adoption. The second is that Meta is one of the first companies to monetise AI. It is already using it for its recommendation engine to improve user engagement, and even to create automated content for advertisers on its platforms. It's early days yet, but we think that gives Meta an edge.
Ready for the future?
We also look into much longer-term innovations like quantum computing, humanoid robots and brain-machine interfaces. Although these are still at least a decade away, making it too early to invest, we are actively watching developments in these areas, where we see huge potential.